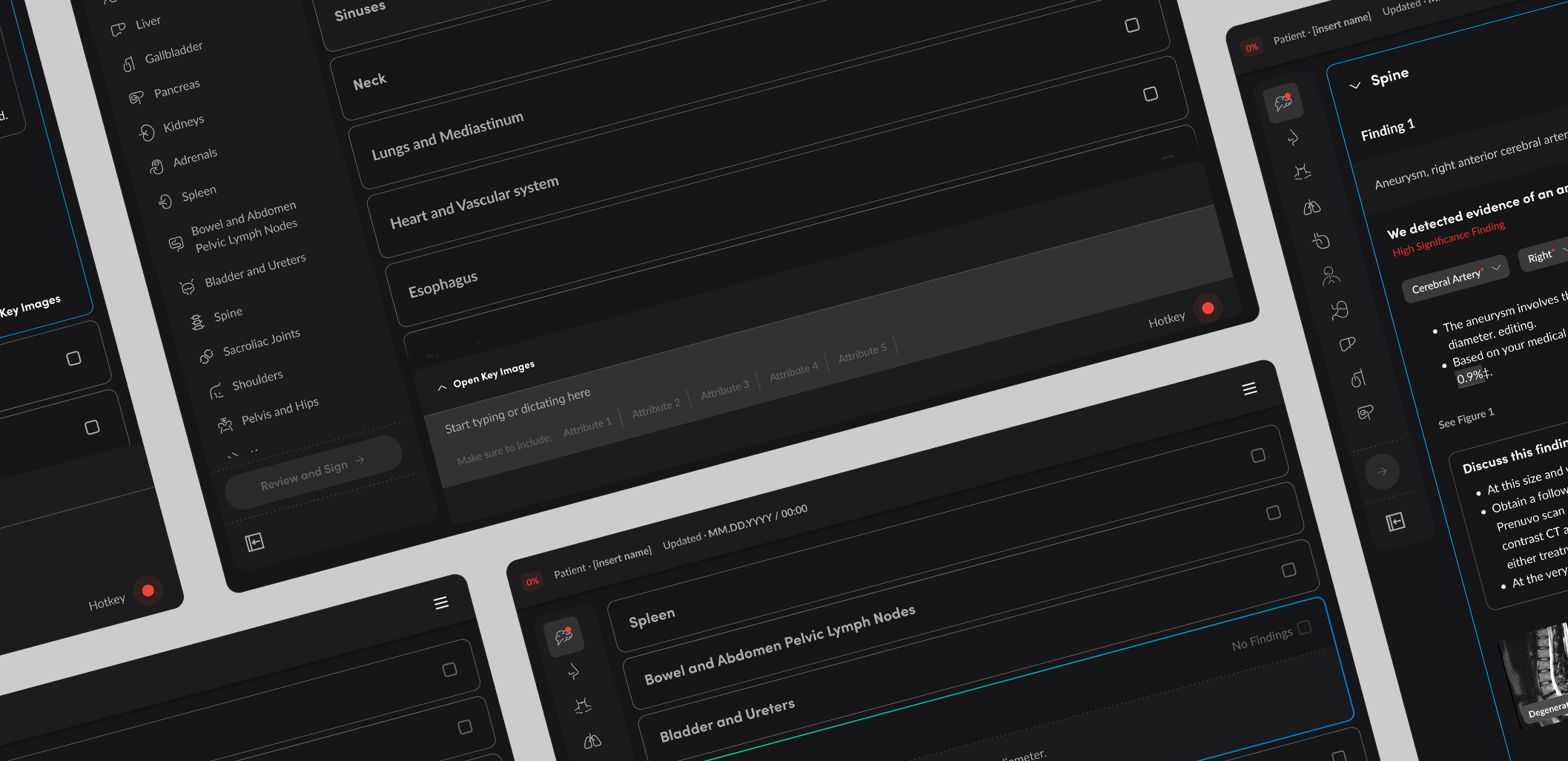
Head of Design
HeathTech
Product Design.
Outcomes
_40% reduction in documentation creation time
_Structured findings in specific hierarchies
_15% reduction in processing time
Methods
_Service Design
_Product Design
_Design Operations
_Design system build
_Design research
Streamlining radiology with AI.
Radiologists face immense pressure to deliver high-quality, life-saving reports quickly, all while interpreting complex medical images. Traditionally, they have relied on methods like typing or dictating their findings to human transcriptionists. Although effective, these methods are time-consuming and prone to errors, which can add to the radiologists' workload and delay diagnoses.
To address these challenges, we considered how a voice-enabled AI dictation tool could streamline the documentation process for radiologists. Our goal was to design a solution that not only enhanced efficiency and improved quality, but also structured data in a way that could enable AI to learn and improve over time. We believed, AI would be well-suited for radiologists, as it eliminated the need to transcriptions to be manually inputed, thus radiologists could focus on interpreting images and delivering insights — rather than on the mechanics of documentation.
Approach
Our approach assumed that modern AI technologies could effectively handle the unique demands of imaging transcription. Specifically, we needed the AI to transcribe complex medical and radiology-specific terminology with a high degree of accuracy. If the AI could achieve this level of performance, it would reduce time spent on documentation review, further improving radiologists' workflows.
Challenge
Our design challenge, then, was twofold. First, we needed to create a voice-enabled interface that radiologists could easily adopt and incorporate into their daily workflow. Secondly, the tool had to reliably deliver accurate transcriptions. By addressing these two needs, we could deliver a tool that streamlined workflows, reduce errors, and improve outcomes.
Designing
We started with in-depth interviews and process shadowing to understand radiologists' workflows and pain-points. These insights, enabled us to draft low-fidelity discreet feature prototypes, which under went multiple rounds of testing and refinement with radiologists. This led to intuitive new features which could be integrated into the existing workflow - without disruption.
Voice recognition and natural language processing (NLP) technologies were integrated incrementally to the hanging protocol (a set of rules that determines how images are displayed on a computer monitor for review). By focusing on single body parts, the tool built context understanding, ultimately matching human transcription quality. By structuring data from transcriptions, we enabled easier extraction and analysis in AI systems, providing almost immediate business value through enhanced intelligence and operational insights.
Customisation options and robust error handling features were added to enhance usability and reliability as the product evolved. Throughout the process, our focus was on minimising the adoption learning curve and ensuring seamless workflow integration. This objective led us to realise that the next version of the product could be radically simplified again iby further studying how radiologists handle each aspect of the hanging protocol.
Product features
Speech recognition When a radiologist speaks into a microphone or headset, the product captures their voice and converts it into text. It also guides the radiologist to structure their findings in specific hierarchies, enabling the business to start to build synthetic radiologist models that will further automate the process.
Contextual understanding The product understands the context in which radiology and medical terms are used, allowing it to accurately transcribe even the most complex jargon. It is trained on a vast lexicon of terms and phrases, ensuring accurate transcription of complex anatomical terms and diagnoses, thus enhancing overall documentation quality.
Patient efficiency The product reduces the time radiologists need to review a patient by using intelligent prompts and patient intelligence to guide them. This allows radiologists to concentrate on interpreting images and making critical decisions rather than being bogged down by cumbersome systems. And allows the business to scan more patients.
Automated documentation The product's accuracy and consistency lead to improved documentation quality. Radiologists can generate detailed, well-structured, and intuitive reports essential for patient care, research, and legal purposes. The product combines key images, findings, and recommendations, formatting them on the as the data capture occurs.
Compliance Patient information remains confidential, and the product is designed to meet the stringent standards of the healthcare industry. It follows best practices in documenting the design process, ensuring compliance with industry regulations.
Results
The Radiologist voice-enabled AI dictation product successfully addressed the key challenges of efficiency, documentation quality, and data structuring. The adoption of a design led approach iterative testing created a tool that significantly enhances the Radiologists' workflow and provides valuable business data.
Estimated 40% reduction in documentation creation time, allowing more focus on image analysis.
User satisfaction increased 30%, with radiologists appreciating ease of use and workflow integration.
Structured data enabled operational insights, and better business decision-making.
My learnings
This experience highlighted the importance of understanding users' motivations and concerns beyond the immediate needs of the business. It reminded me that product adoption requires addressing not only the functional requirements but the human elements of change and the impact AI could have on all our careers. Despite the success for the business, I recognise there's still much to learn, particularly in the areas of AI and user interface design.
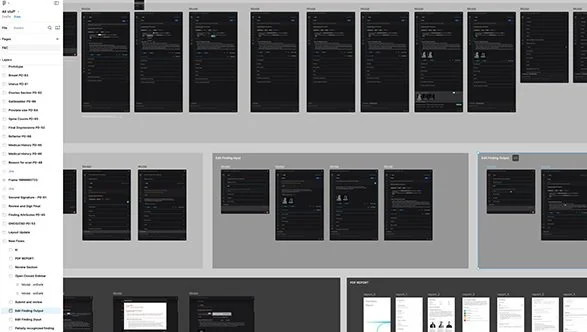


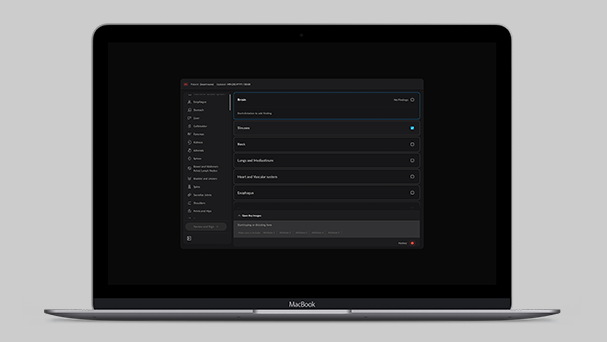

